Generative AI meets Enterprise Software
“Gradually, then suddenly” - Hemingway. That’s been our journey with AI through the years, and the promise is finally being fulfilled. Like cloud software defined the last two decades, this massive shift will be at the center of enterprise software innovation for the next decade.
The speed of innovation is equal parts exciting and anxiety-inducing, both to founders and investors. We've been spending time with B2B founders and domain experts and have made several investments in the sector. This is our early take on opportunities for founders to rethink enterprise SaaS with an AI-first lens.
- Don't build a faster horse.
Many people call ChatGPT the iPhone moment for AI, so let’s retrace history. The iPhone was launched in 2007, and the Appstore followed a year later in 2008. This spurred all web-first companies to launch mobile apps, and Facebook, Amazon, etc., followed suit with mobile apps. This was incremental innovation, going from web to web+mobile. The mobile-native era was really ushered in by companies like Uber (2010), Snapchat (2010), and even Pokemon Go (as late as 2016). These experiences were designed ground up for the smartphone, putting GPS and/or cameras at the core of the experience, and could not be replicated on a desktop.
The first phase of B2B generative AI applications draws a similar pattern - they are add-ons to existing workflows. Copilots-for-everything. Incremental innovation makes incumbents stronger. The cloud wave had a lot of friction for incumbents when moving, including rearchitecting for multi-tenancy, solving performance issues, and more. Still, this shift is easier to navigate with easy plug-and-play APIs. They already have the workflows, the datasets, the distribution, and can consume APIs of foundational models to ship features fast. See the recent releases from Intercom, Ironclad, Github, Replit, etc., and you’ll get the drift.
We believe that the true power of enterprise AI will be unlocked when we reimagine business workflows with AI-nativity vs. AI as a layer. Just like Canva (Matrix portfolio company) made everyone a great designer, genAI is democratizing a lot of hard skills (writing, coding, design) and there will be a lot of change in how functional teams work together. And we are really early in the journey of rebuilding user workflows from the ground up, or in imagining how many verticals will have wholesale changes in how they operate.
- Rethink UX with AI at the core.
One place where startups can outpace incumbents who will struggle to move fast is UX innovation. Imagine a world where every sales rep, instead of looking at tables of leads and picking the next lead to work on, is instead looking at a playlist of tasks generated and prioritized by AI. Going one step further, genAI writes scripts for outgoing communications such as call notes, follow-ups, welcome messages, etc. When it comes to these actions, the sales rep can move from creator to auditor. Instead of a rep working for a CRM, the CRM might actually work for the sales rep!
Look at two examples to design creatives: Microsoft Designer and Adobe Fireflies.
One targets low levels of expertise (my parents, even!), and the other targets experts who want creative control. But instead of leaving it to creative prompt engineering, Adobe presents the options as a menu of options that mimics a creative’s thought process. We’ve seen chat as the first UX, but chat is just one among many. We expect a lot of UX innovation from companies that help users get from idea to outcome with opinionated workflows, AI embedded in the experience, and working behind the scenes.
- AI+People business models
Enterprises like predictability and generative AI offers anything but. In the previous era of AI used for understanding language, there was only one right version of the answer, and technology converged toward this answer. But when it comes to generation, trusting output is hard because models can diverge with answers, and this gets amplified at scale. Explaining your reliability to enterprises is even harder.
The market is early, and enterprise buyers are uncomfortable with genAI software in product use cases, especially when it comes to customer-facing workflows. Startups can innovate by moving away from pure SaaS plays to software + people offerings to calm the nerves of enterprise buyers.
- Blackbox AI+People - When Expensify launched, we got an app to scan invoices and convert them into expense reports. We didn’t know that behind the scenes, 3 out of 10 invoices were parsed by people. We know that GenAI model outputs are probabilistic, not absolute. Enterprises want accuracy and reliability, and one way to offer this is to package AI + services as a black box. They get a service level guarantee, you get operational leverage with AI automating 80%, and services team auditing flows and handling exceptions. These models will be more common in high-accuracy use cases like document parsing for invoices, medical coding, legal, etc. Partnering with system integrators might also create a distribution edge where they sell your software and manage the services.
- Software + Talent Marketplaces - Our portfolio company, Murf.ai, develops synthetic speech, and found its first clients on Upwork and Fiverr. Buyers were ok with AI-generated speech once they heard the quality, and this was a telling moment for us. Enterprise buyers care about quality and speed of outcomes; how these are delivered is second nature. Startups can blur the lines of what is delivered by AI vs. humans. Use AI for quality levels 1-6, get an auditor for levels 6-8, or hire an expert for quality 9-10.
- Professional services as a moat - offer a team to work with the enterprise to suggest best-practice deployments, fine-tune models, and get engrained in the enterprise.
Till enterprise buyers build comfort and confidence with AI deployments at scale, we believe the services element will be an important factor in enterprise buying decisions. Founders building from India to the globe can leverage this to get ahead and deploy our pools of rich IT services talent to innovate on their offerings and GTM motions.
- Build an edge with data
We believe that data is a quality game vs. a quantity game. RLHF can get you to high-quality results with smaller datasets, provided they are the right ones. Once you land in an enterprise with a killer workflow, you see how users consume GenAI outputs - what do they accept, edit, and reject? This data can be used to finetune models for broad use cases or train smaller language models (SLMs) for narrow use cases and make gains on cost, quality, and speed. Keeper Tax, a Matrix portfolio company, has done exactly this. The product helps freelancers identify business expenses in their statements and save taxes. As freelancers use the product, they’ve built a data edge in reading bank and credit card statements and understanding which expenses are business vs. personal for specific types of freelancers.
This data advantage can help you get a leg up on quality metrics and further strengthen your ROI for the enterprise. Data may or may not be a moat in the long term, but it can help you build a headstart, land with new customers using your workflow as a wedge, and buy time to build deeper workflows.
We also believe that switching costs for AI-native solutions are higher. At my past company, we used a lead scoring system, and we trusted it so much that our marketing goals were set on machine-qualified leads (a different type of MQL). This system became a neutral auditor between marketing and sales, and we found it very hard to switch out because of the team’s inherent trust in the system. Playing this pattern out - once customers come on board and trust your system, they are less likely to move to another platform with unknown performance characteristics. Category churn for AI-native companies can be lower than traditional SaaS benchmarks.
Data privacy and ethics will play an important role in how enterprises make buying decisions, and founders should consider these choices early in the journey. Enterprises have to make hard choices on what data is core to their company, whether it should be shared with vendors and models, how it is used for training and fine-tuning, etc. This should inform early decisions on what datasets you ask for, how you use the data, and whether you use open-source LLMs or one of the large foundational models like GPT-4 or Bard.
- LLMOps leaves a lot to be desired.
Deploying LLMs for B2B use cases is challenging. Despite the noise, we've seen very few AI-first products in production and at scale.
If we go back to the cloud era, many tools and platforms were built to help developers ship cloud products at scale and with reliability. When it comes to GenAI, the canvas is still wide open. Just take the example of testing - in the past, there was one "correct answer", and testing tools had to compare 1s and 0s. In the world of LLMs, we need a new testing paradigm that compares multiple versions of text for accuracy and prose.
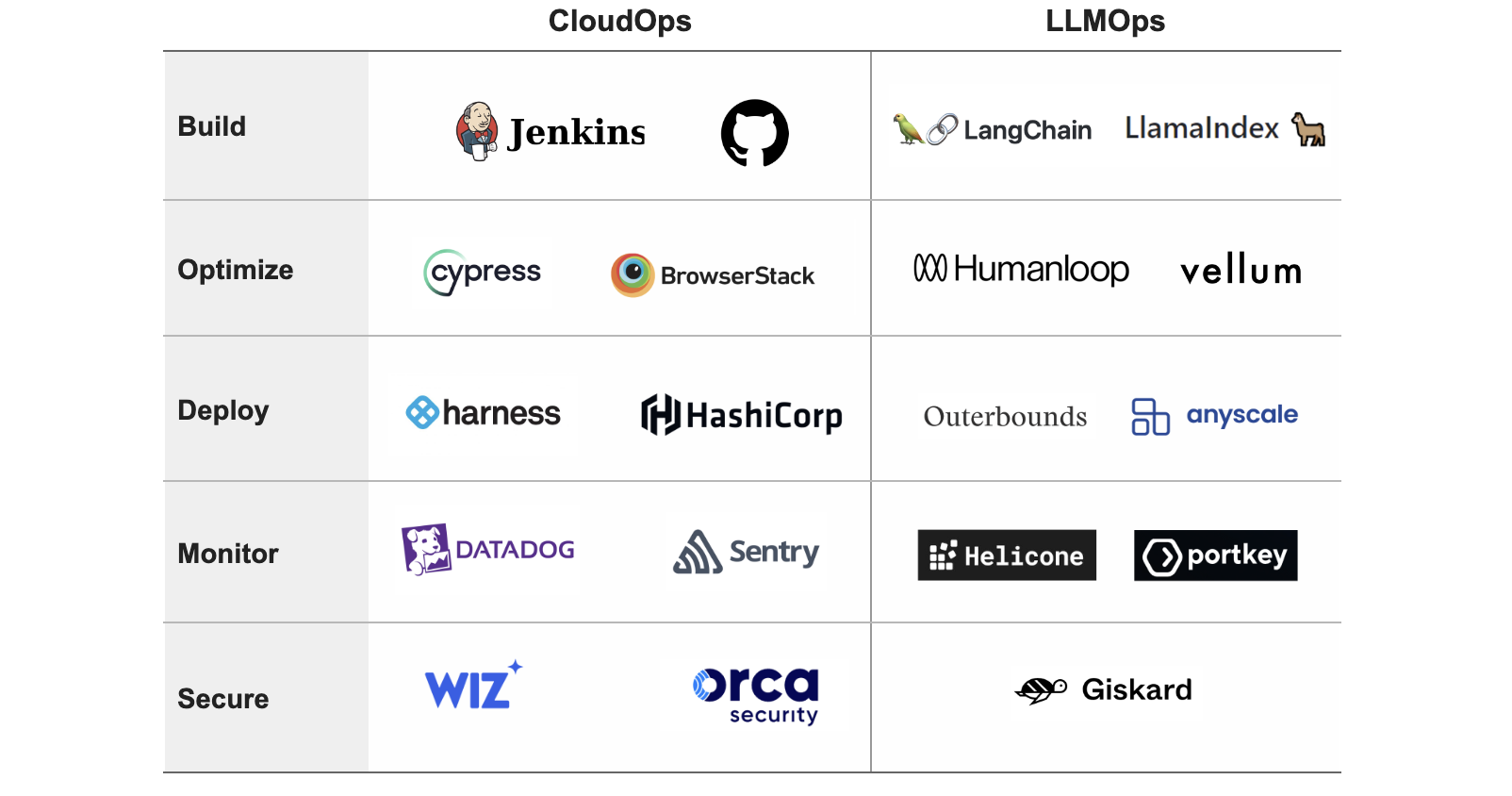
There are many enterprise problems, such as monitoring models for quality and drift, explaining and auditing models, and ensuring a strong privacy and compliance posture so that sensitive data is not pushed to third parties or used in training and committed to memory. Boring enterprise workflows are the foundations for many large companies, and this opportunity is wide open for founders to solve.
In conclusion
GenAI will define the next decade of enterprise software innovation, and we’re excited by the opportunity to build global enterprise AI startups from India. There are interesting wedges to land with enterprises and compete against lazy incumbents, an early-mover advantage to build a lead on data, and, ultimately, a window of opportunity to create the next generation of software that powers the modern enterprise.
As a quick recap, we think that product and business strategy should revolve around the following:
1. Build with an AI-native strategy
2. Find a killer workflow with customer backward thinking
3. Innovate on the business model with an AI+people approach
4. Leverage data to build your edge
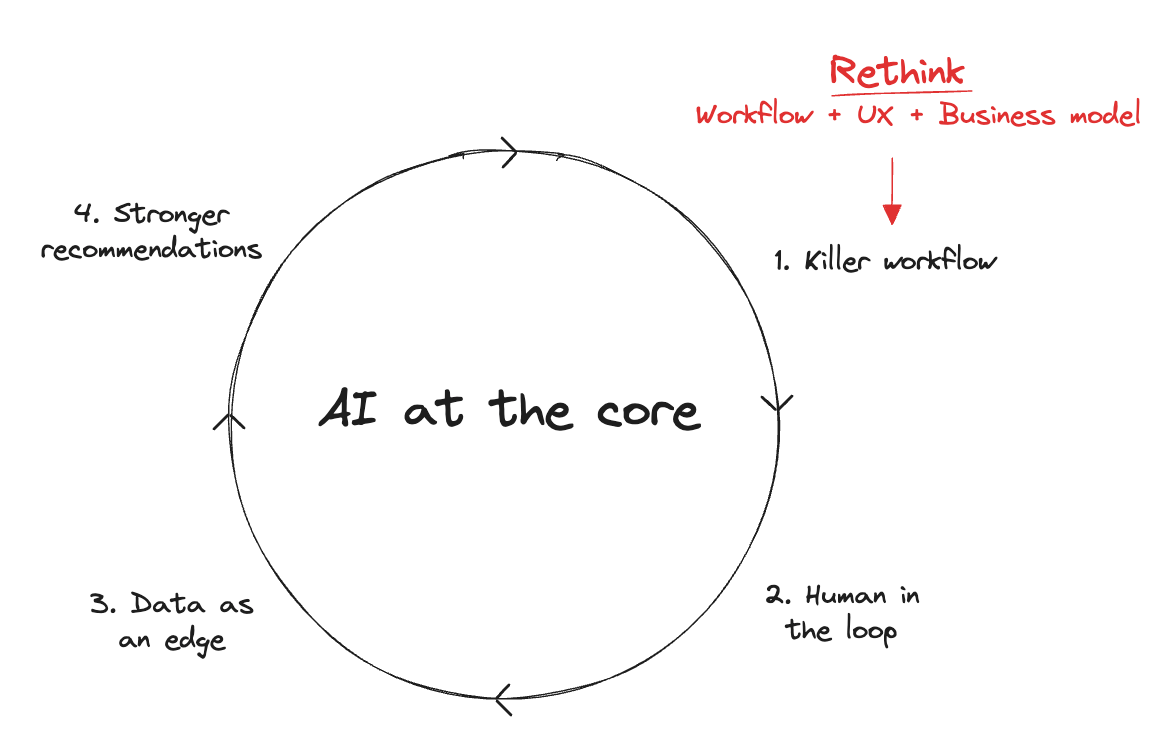
Our objective is to learn together as the sector matures, and we’ve been meeting several people in the domain. We also crowdsource and maintain a repo of GenAI startups from India. If you are building AI-native B2B startups, we would love to whiteboard ideas with you. Write to us at [email protected] or [email protected].
And a big thank you to the experts who helped us with their perspectives - Sundeep Teki, Ayush Garg, Vivek Ys, Debdoot Mukherjee, Ramesh Parthasarathy, Rohit Agarwal, Ankur Edkie, Vijay Rayapati, Soumyadeep Mukherjee, Manisha Raisinghani, Anoop Dawar, Parasvil Patel.